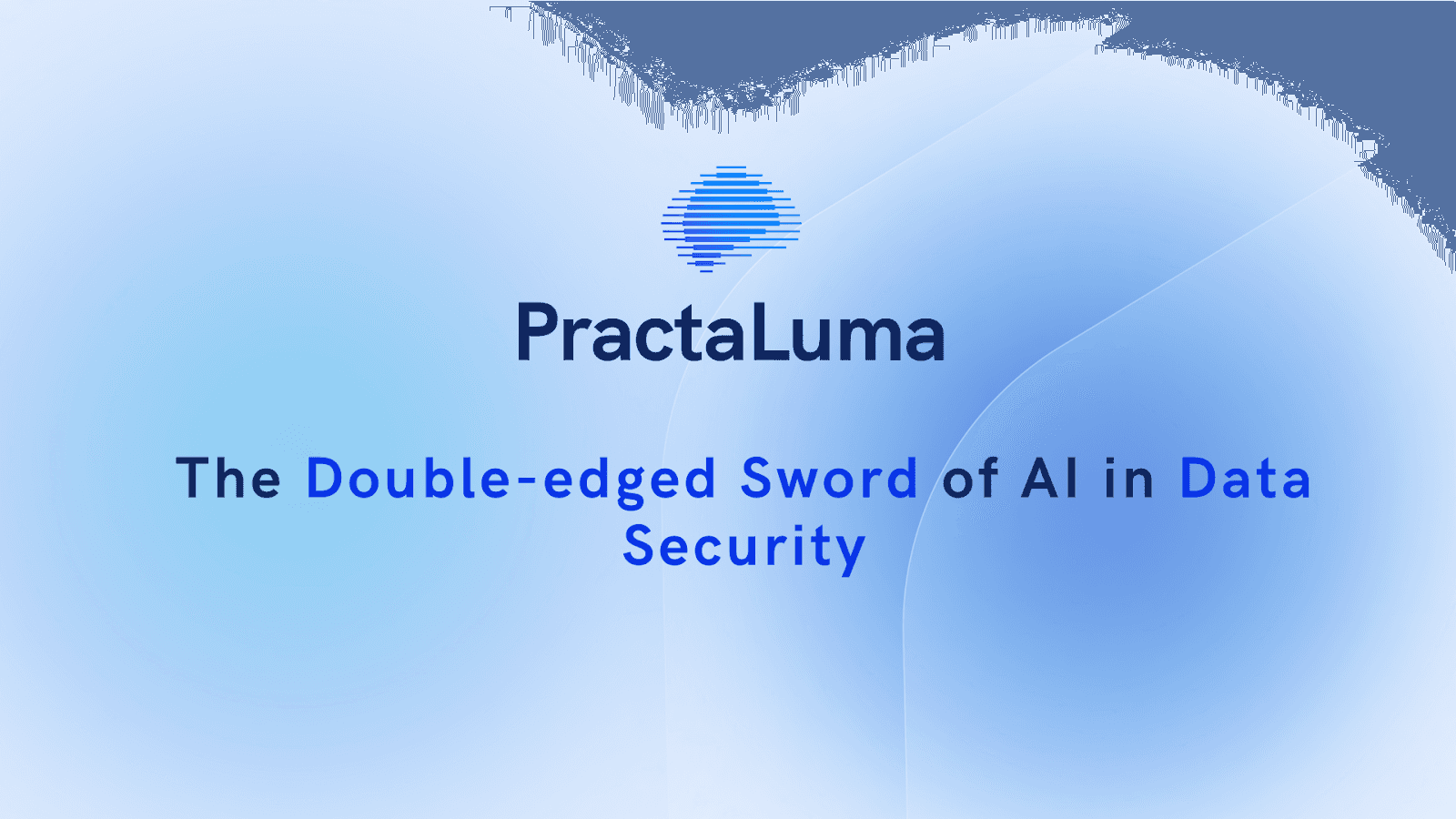
5 May 2025
The Double-edged Sword of AI in Data Security
Artificial Intelligence (AI) is reshaping industries globally, and the mental health sector in Australia is no exception. As healthcare organisations increasingly rely on digital systems, ensuring robust data security has become a pressing concern for mental health practitioners.
While AI offers powerful tools to enhance data protection, it also introduces new risks that must be critically assessed to safeguard sensitive patient information.
According to a 2024 YouGov study, 82% of Australians are concerned about the security of their personal health records. Any breach compromises patient confidentiality, undermines trust, and disrupts the therapeutic relationship—a cornerstone of mental health care. As AI technologies become more prevalent, mental health practitioners must navigate the dual challenge of utilising these tools to enhance security while addressing associated vulnerabilities.
What is the Dual Role of AI in Cybersecurity?
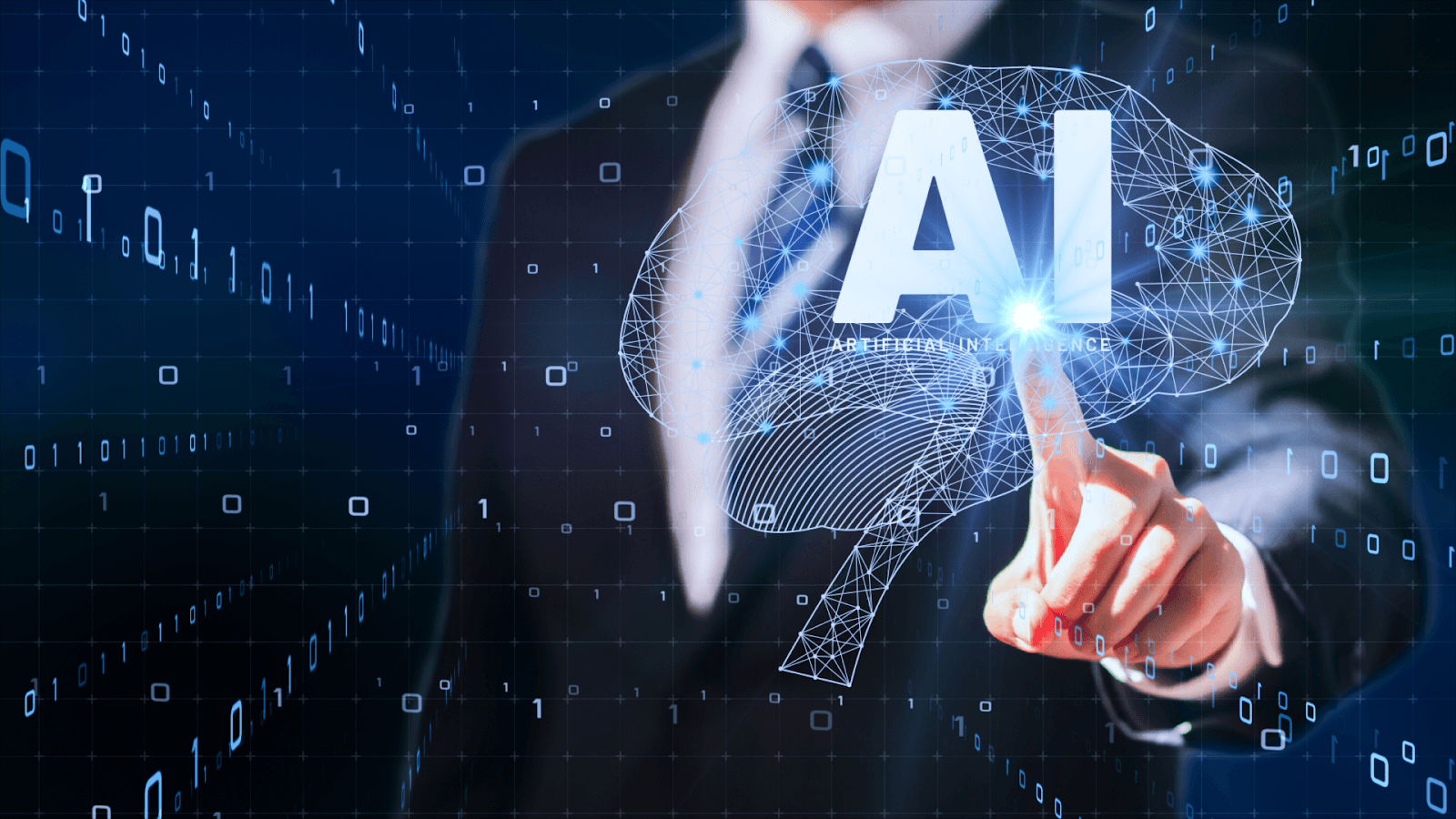
AI has emerged as both a saviour and a potential risk in cybersecurity. Machine learning algorithms and advanced analytics offer unprecedented capabilities to detect, prevent, and mitigate security challenges. However, malicious actors are exploiting the same technologies to conduct sophisticated cyberattacks.
For mental health professionals in Australia, understanding this duality is crucial to implementing AI solutions responsibly to improve their patient care and practice.
What are the AI Applications in Data Security for Mental Health Practitioners?
AI-driven threat detection systems provide mental health organisations with adaptive, intelligent defences that anticipate and neutralise cyber risks. These tools offer key features such as:
- Real-time Threat Identification: Rapidly detecting potential breaches as they occur.
- Pattern Recognition: Identifying suspicious behaviours and anomalies beyond human capability.
- Predictive Analysis: Proactively flagging potential threats based on historical trends.
By integrating machine learning algorithms and behavioural analysis models, AI enhances an organisation’s ability to respond swiftly to emerging threats.
Advanced Threat Detection
Traditional methods of authentication are inadequate in today’s digital landscape. AI-powered systems revolutionise identity management by offering intelligent and adaptive solutions such as:
- Biometric Recognition: Using fingerprints, facial recognition, or voice patterns for secure access.
- Contextual Login Analysis: Evaluating the context of access attempts, such as geographic location or device type.
- Continuous Authentication: Monitoring user activity to ensure ongoing verification.
These capabilities are particularly important for mental health practitioners who handle highly sensitive patient data, including records accessed remotely via telehealth platforms. Similarly, identity and access management systems play a critical role in safeguarding sensitive data by verifying user identities, controlling system access, and much more. Let’s take a closer look at identity and access management below.
What is Identity and Access Management?
AI can also transform vulnerability management from a reactive process to a proactive security strategy. Key features include:
- Comprehensive System Scanning: Identifying vulnerabilities across all digital assets.
- Intelligent Risk Prioritisation: Using AI to focus on the most critical vulnerabilities.
- Automated Patching: Deploying patches quickly to minimise exposure to threats.
These automated processes reduce human error and ensure mental health organisations maintain robust security protocols.
Benefits of AI in Data Security
For mental health practitioners practicing telemedicine, the benefits of AI in data security are profound. Key advantages include:
- Enhanced Compliance: AI tools help organisations adhere to Australian Privacy Principles (APPs) and other regulatory requirements.
- Preservation of Trust: By protecting sensitive patient data, mental health practitioners can maintain trust and confidentiality, which are essential components of therapeutic relationships.
- Scalability: AI systems can handle increasing volumes of data and users without compromising security, making them ideal for growing telemedicine practices.
- Cost Efficiency: Automating security processes reduces reliance on extensive manual oversight, lowering operational costs while maintaining robust protection.
- Real-Time Monitoring: Continuous system surveillance allows for instant detection and mitigation of security threats.
- Behavioural Analytics: AI can identify unusual patterns of behaviour to flag potential breaches or insider threats.
While AI presents many promising solutions, it also introduces unique risks that mental health professionals must understand and address. Hence, known as the dual-edge sword! Let’s find out the threats of AI.
Threats of AI in Data Security
AI can threaten data security in the following ways:
1. Adversarial Attacks
Malicious actors can manipulate AI models to produce incorrect or harmful outputs. For example, adversarial attacks can:
- Exploit subtle vulnerabilities in machine learning algorithms.
- Disrupt critical decision-making systems, such as biometric authentication.
- Target telehealth platforms, compromising patient confidentiality.
2. Model Inversion Attacks
Cybercriminals can reverse-engineer AI models to retrieve sensitive information from the training data. For instance:
- Exposing Patient Records: If AI is trained on unprotected medical data, attackers can exploit it to uncover patient details.
- Sensitive Insights at Risk: Even anonymised data can sometimes be reconstructed to reveal personal identities, violating privacy regulations.
3. Data Poisoning
Attackers can deliberately introduce corrupted or misleading data into AI training datasets, compromising the reliability of the system. For example:
- Compromised Predictions: Poisoned data can lead to inaccurate detection or misclassification of threats.
- Operational Risks: Such tampered models could undermine trust in automated systems and disrupt security protocols.
4. Algorithmic Misuse
AI tools can be weaponised by malicious actors to automate and amplify cyberattacks. Examples include:
- Scalable Phishing Campaigns: AI can generate highly personalised phishing emails at scale, making attacks harder to detect.
- Automated Malware Deployment: Sophisticated AI algorithms can craft and deploy malware that adapts to evade traditional security measures.
5. Over-reliance on AI
Organisations might overly depend on AI-driven systems without adequate human oversight, which could lead to:
- Undetected Vulnerabilities: Relying solely on AI may miss complex or evolving threats that require human intuition to identify.
- Crisis Handling Gaps: In situations where AI systems fail or are targeted, the lack of manual backup processes can exacerbate security breaches.
Such breaches can erode trust in mental health services and contravene Australia’s Privacy Act and the Australian Privacy Principles (APPs). But even with that, the risks don’t end there.
AI learns from humans, which causes it to learn the same biases as humans. It is important to know these biases present so that they can be caught before they damage your relationships with your patients.
Common Bias in AI Models
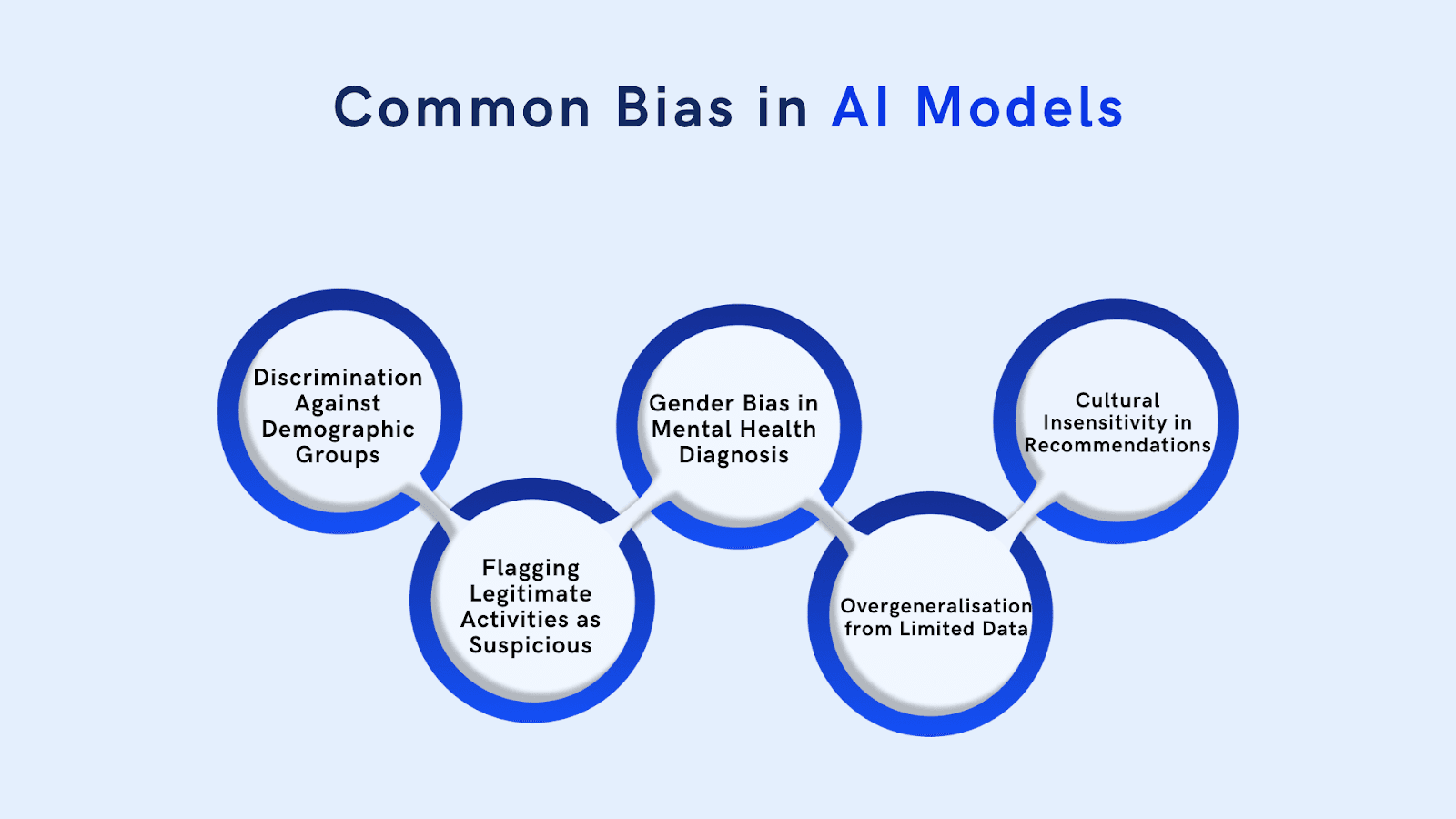
AI algorithms can unintentionally reinforce biases in their training data, raising ethical concerns in mental health and therapy. These biases often stem from unrepresentative datasets or societal inequities, affecting patient outcomes and the therapeutic relationship. Common issues include:
- Demographic Discrimination: AI may underdiagnose mental health conditions in minority groups due to limited representation, leading to misdiagnoses or inadequate care.
- Misclassification of Behaviour: AI tools may wrongly flag behaviours like frequent telehealth use as high-risk, straining trust between patients and therapists.
- Gender Bias in Diagnosis: AI may reinforce stereotypes, such as overdiagnosing anxiety in women or underestimating depression in men, resulting in ineffective treatment.
- Overgeneralisation: AI trained on urban data may misinterpret mental health needs in rural populations, limiting access to accurate care.
- Cultural Insensitivity: AI may overlook cultural or religious factors in treatment plans, making recommendations feel irrelevant or alienating.
These biases threaten fairness in mental health care, but they can be managed with careful oversight and diverse, representative data. Let’s explore how to safeguard your practice from these risks.
How to Manage Threat and Response with AI?
Mental health organisations are increasingly using AI-driven strategies to tackle evolving cyber threats. These strategies offer several key benefits:
- Automating Threat Management: AI streamlines security processes by detecting threats in real-time, automating incident responses, and continuously monitoring systems to prevent breaches.
- Prioritising Risks & Responses: AI assesses vulnerabilities, automates patch management, and adapts security measures to emerging threats, strengthening overall protection.
- Enhancing Data Security: AI-powered encryption secures sensitive data during transmission and storage, while AI-driven systems ensure secure communication channels and detect phishing attempts.
- Simulating Cyber Threats: AI enables threat simulations, staff training, and system stress testing to improve preparedness and resilience against attacks.
- Predictive Threat Analytics: AI analyses historical patterns to predict cyber threats, allowing for early warnings, proactive countermeasures, and long-term security improvements.
- Tailored Security for Mental Health Practices: AI protects telehealth platforms, ensures patient confidentiality, and helps organisations comply with Australian privacy regulations.
By leveraging AI, mental health organisations can enhance security, protect patient data, and stay ahead of cyber threats.
Conclusion
For mental health practitioners in Australia, AI represents both an opportunity and a challenge in safeguarding patient data. Organisations can better protect sensitive information and maintain trust by employing advanced AI-driven security tools. However, the potential risks associated with AI—such as adversarial attacks, data leakage, and bias—require proactive management and ethical oversight.
The future of mental health data protection lies in striking a balance: embracing AI’s transformative potential while upholding the ethical principles and trust that form the foundation of effective care. By taking a thoughtful, proactive approach, mental health professionals can ensure that technology serves as an ally in delivering secure, equitable, and high-quality care to their patients.
This is where PractaLuma’s AI Clinical Assistant stands out. Its secured data storage and threat management capabilities make it a perfect fit for you to integrate into your practice and increase the time dedicated to patient care. To know more, check out PractaLuma here.
Frequently Asked Questions (FAQs)
1. How does AI enhance data security for mental health practitioners?
AI enhances data security by offering real-time threat detection, pattern recognition, and predictive analysis. These capabilities allow mental health organisations to swiftly identify and mitigate potential cyber risks, protecting sensitive patient information.
2. What are the primary cybersecurity risks associated with AI in mental health care?
Key risks include adversarial attacks that exploit AI vulnerabilities, data leakage due to inadequate anonymisation or access controls, and biases in AI models that can lead to ethical and operational challenges.
3. How can AI-driven tools ensure compliance with Australian Privacy Principles (APPs)?
AI tools help organisations maintain compliance by automating data security processes like vulnerability scanning, patch management, and access control. These tools also monitor for breaches, ensuring timely responses to maintain privacy standards.
4. What measures can mental health practitioners take to mitigate AI-related biases?
Practitioners can mitigate biases by:
- Regularly auditing AI systems for fairness and inclusivity.
- Ensuring diverse, unbiased training datasets.
- Engaging in continuous education about AI ethics and potential biases.
5. How does AI improve identity and access management for mental health organisations?
AI-driven solutions enhance identity and access management through advanced methods like biometric recognition, contextual login analysis, and continuous authentication. These measures provide robust security, particularly for telehealth platforms.
6. What makes PractaLuma’s AI Clinical Assistant a good fit for mental health practitioners?
PractaLuma’s AI Clinical Assistant offers secure data storage, advanced threat management, and automation capabilities. These features enable practitioners to focus on patient care while ensuring their data remains protected.
7. Can AI eliminate cybersecurity risks for mental health practitioners?
While AI significantly reduces cybersecurity risks, it cannot eliminate them entirely. A comprehensive security strategy combining AI tools with proactive human oversight, regular updates, and ethical considerations is essential for optimal protection.